Training Doppler Radar With Smart Watch IMUs Data For Activity Recognition
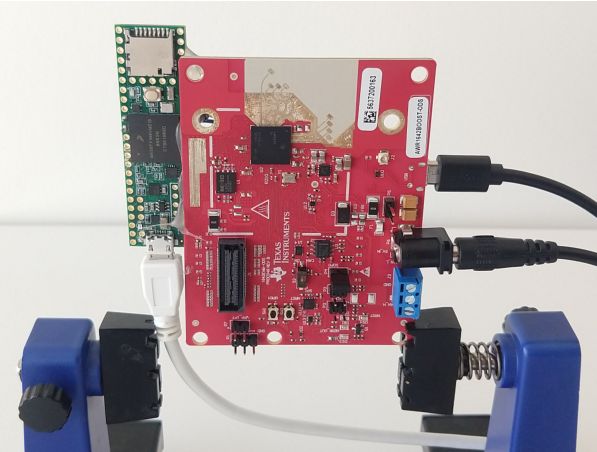
When it comes to interpreting sensor data automatically, it helps to have a large data set to assist in validating it, as well as training when it concerns machine learning (ML). Creating this data set with carefully tagged and categorized information is a long and tedious process, which is where the idea of cross-domain translations come into play, as in the case of using millimeter wave (mmWave) radar sensors to recognize activity of e.g. building occupants with the IMU2Doppler project at Smash Lab of Carnegie Mellon University.
The most commonly used sensor type when it comes to classifying especially human motion are inertial measurement units (IMU) such as accelerometers and gyroscopes, which are found in everything from smartphones to smart watches and fitness bands. For these devices it’s common to classify measurement patterns as matches a particular activity, such as walking, jogging, or brushing one’s teeth. This makes them both well-defined and very accessible.
As for why a mmWave-based Doppler radar would be preferred for monitoring e.g. building occupants is the privacy aspect compared to using cameras, and the inconvenience of equipping people with a body-worn IMU. Using Doppler radar it would theoretically be possible for people to track activities within their own home, as well as in a medical setting to ensure patients are safe, or at a gym to track one’s performance, or usage of equipment. All without the use of cameras or personal sensors. In the past, we’ve seen a similar approach that used targeted laser beams.
As promising as this sounds, at this point in time the number of activities that are recognized with reasonable accuracy (~70%) is limited to ten types. Depending on the intended application this may already be sufficient, though as the published paper notes, there is still a lot of room for growth.
from Blog – Hackaday https://ift.tt/ckYXOv2
Comments
Post a Comment